Overview
The HITS (Hyperlink-Induced Topic Search) algorithm was developed by L.M. Kleinberg in 1999 with the purpose of improving the quality of search methods on the World Wide Web (WWW). HITS makes use of the mutual reinforcing relationship between authorities and hubs to evaluate and rank a set of linked entities.
- L.M. Kleinberg, Authoritative Sources in a Hyperlinked Environment (1999)
Concepts
Authority and Hub
In WWW, hyperlinks represent some latent human judgment: the creator of page p, by including a link to page q, has in some measure conferred authority on q. Instructively, a node with large in-degree is viewed as an authority.
If a node points to considerable number of authoritative nodes, it is referred to as a hub.
As illustrated in the graph below, red nodes are good authorities, green nodes are good hubs.
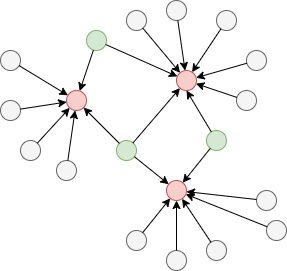
Hubs and authorities exhibit what could be called a mutually reinforcing relationship: a good hub points to many good authorities; a good authority is pointed to by many good hubs.
Compute Authorities and Hubs
HITS algorithm operates on the whole graph iteratively to compute the authority weight (denoted as x) and hub weight (denoted as y) for each node through the link structure. Nodes with larger x-values and y-values are viewed as better authorities and hubs respectively.
In a directed graph G = (V, E), all nodes are initialized with x = 1 and y = 1. In each iteration, for each node p ∈ V, update its x and y values as follows:
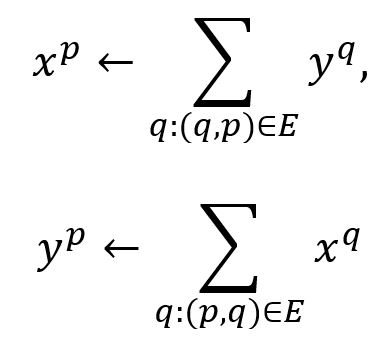
Here is an example:
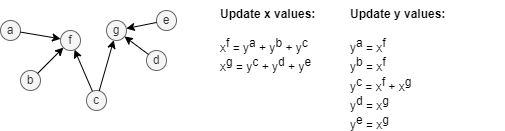
At the end of one iteration, normalize all x values and all y values to meet the invariant below:
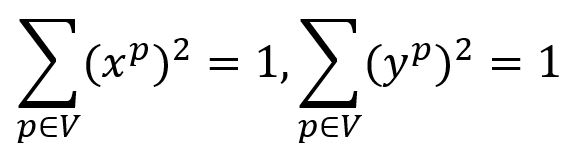
The algorithm continues until the change of all x values and y values converges to within some tolerance, or the maximum iteration rounds is met. In the experiments of the original author, the convergence is quite rapid, 20 iterations are normally sufficient.
Considerations
- In HITS algorithm, self-loops are ignored.
- Authority weight of nodes with no in-links is 0, hub weight of nodes with out-links is 0.
Example Graph
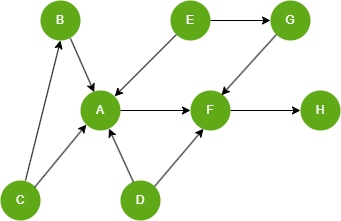
To create this graph:
// Runs each row separately in order in an empty graphset
insert().into(@default).nodes([{_id:"A"}, {_id:"B"}, {_id:"C"}, {_id:"D"}, {_id:"E"}, {_id:"F"}, {_id:"G"}, {_id:"H"}])
insert().into(@default).edges([{_from:"C", _to:"A"}, {_from:"C", _to:"B"}, {_from:"B", _to:"A"}, {_from:"E", _to:"A"}, {_from:"E", _to:"G"}, {_from:"A", _to:"F"}, {_from:"D", _to:"A"}, {_from:"D", _to:"F"}, {_from:"F", _to:"H"}, {_from:"G", _to:"F"}])
Creating HDC Graph
To load the entire graph to the HDC server hdc-server-1
as hdc_hits
:
CALL hdc.graph.create("hdc-server-1", "hdc_hits", {
nodes: {"*": ["*"]},
edges: {"*": ["*"]},
direction: "undirected",
load_id: true,
update: "static",
query: "query",
default: false
})
hdc.graph.create("hdc_hits", {
nodes: {"*": ["*"]},
edges: {"*": ["*"]},
direction: "undirected",
load_id: true,
update: "static",
query: "query",
default: false
}).to("hdc-server-1")
Parameters
Algorithm name: hits_centrality
Name |
Type |
Spec |
Default |
Optional |
Description |
---|---|---|---|---|---|
max_loop_num |
Integer | ≥1 | 20 |
Yes | The maximum number of iteration rounds. The algorithm will terminate after completing all rounds. |
tolerance |
Float | (0,1) | 0.001 |
Yes | The algorithm terminates when the changes in all authority and hub weights between iterations are less than the specified tolerance , indicating that the result is stable. |
return_id_uuid |
String | uuid , id , both |
uuid |
Yes | Includes _uuid , _id , or both values to represent nodes in the results. |
limit |
Integer | ≥-1 | -1 |
Yes | Limits the number of results returned; -1 includes all results. |
File Writeback
CALL algo.hits_centrality.write("hdc_hits", {
params: {
return_id_uuid: "id"
},
return_params: {
file: {
filename: "ranks"
}
}
})
algo(hits_centrality).params({
return_id_uuid: "id",
projection: "hdc_hits"
}).write({
file: {
filename: "ranks"
}
})
Result:
_id,authority,hub
D,0,0.572083
F,0.42642,1.43197e-11
H,3.20199e-11,0
B,0.213196,0.381382
A,0.852796,0.190701
E,0,0.476726
C,0,0.476726
G,0.213196,0.190701
DB Writeback
Writes the authority
and hub
values from the results to the specified node property. The property types are both double
.
CALL algo.hits_centrality.write("hdc_hits", {
params: {
max_loop_num: 20,
tolerance: 0.0001
},
return_params: {
db: {
authority: 'auth',
hub: 'hub'
}
}
})
algo(hits_centrality).params({
projection: "hdc_hits",
max_loop_num: 20,
tolerance: 0.0001
}).write({
db: {
authority: 'auth',
hub: 'hub'
}
})
Full Return
CALL algo.hits_centrality("hdc_hits", {
params: {
return_id_uuid: "id"
},
return_params: {}
}) YIELD r
RETURN r
exec{
algo(hits_centrality).params({
return_id_uuid: "id"
}) as r
return r
} on hdc_hits
Result:
_id | authority | hub |
---|---|---|
D | 0 | 0.572083 |
F | 0.42642 | 0 |
H | 0 | 0 |
B | 0.213196 | 0.381382 |
A | 0.852796 | 0.190701 |
E | 0 | 0.476726 |
C | 0 | 0.476726 |
G | 0.213196 | 0.190701 |
Stream Return
CALL algo.hits_centrality("hdc_hits", {
params: {
return_id_uuid: "id",
max_loop_num: 20,
tolerance: 0.0001
},
return_params: {
stream: {}
}
}) YIELD r
RETURN r._id, r.hub ORDER BY r.hub DESC
exec{
algo(hits_centrality).params({
return_id_uuid: "id",
max_loop_num: 20,
tolerance: 0.0001
}).stream() as r
return r._id, r.hub order by r.hub
} on hdc_hits
Result:
r._id | r.hub |
---|---|
D | 0.572083 |
E | 0.476726 |
C | 0.476726 |
B | 0.381382 |
A | 0.190701 |
G | 0.190701 |
F | 0 |
H | 0 |