Overview
Jaccard similarity, or Jaccard index, was proposed by Paul Jaccard in 1901. It’s a metric of similarity for two sets of data. In the graph, collecting the neighbors of a node into a set, two nodes are considered similar if their neighborhood sets are similar.
Jaccard similarity ranges from 0 to 1; 1 means that two sets are exactly the same, 0 means that the two sets do not have any element in common.
Concepts
Jaccard Similarity
Given two sets A and B, the Jaccard similarity between them is computed as:

In the following example, set A = {b,c,e,f,g}, set B = {a,d,b,g}, their intersection A⋂B = {b,g}, their union A⋃B = {a,b,c,d,e,f,g}, hence the Jaccard similarity between A and B is 2 / 7 = 0.285714
.
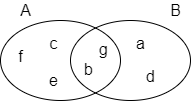
When applying Jaccard Similarity to compare two nodes in a graph, we use the 1-hop neighborhood set to represent each target node. The 1-hop neighborhood set:
- contains no repeated nodes;
- excludes the two target nodes.
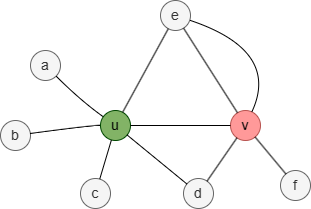
In this graph, the 1-hop neighborhood set of nodes u and v is:
- Nu = {a,b,c,d,e}
- Nv = {d,e,f}
Therefore, the Jaccard similarity between nodes u and v is 2 / 6 = 0.333333
.
In practice, you may need to convert some node properties into node schemas in order to calculate the similarity index that is based on common neighbors, just as the Jaccard Similarity. For instance, when considering the similarity between two applications, information like phone number, email, device IP, etc. of the application might have been stored as properties of @application node schema; they need to be designed as nodes and incorporated into the graph in order to be used for comparison.
Weighted Jaccard Similarity
The Weighted Jaccard Similarity is an extension of the classic Jaccard Similarity that takes into account the weights associated with elements in the sets being compared.
The formula for Weighted Jaccard Similarity is given by:
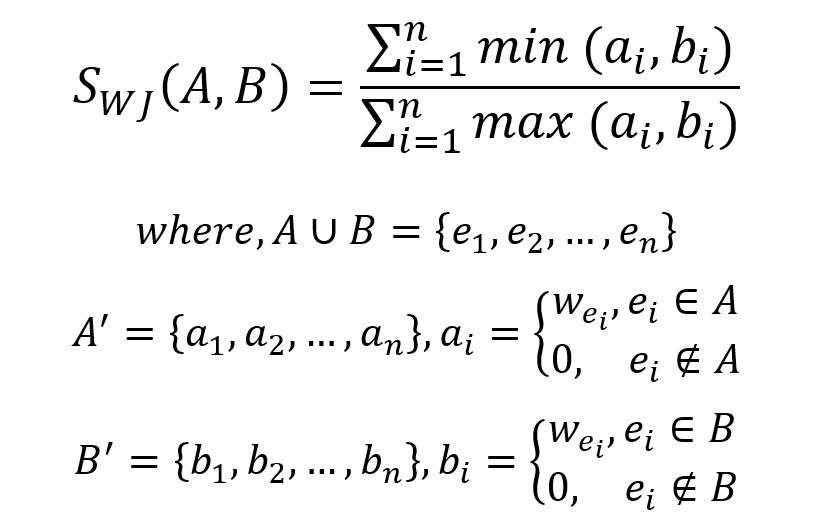
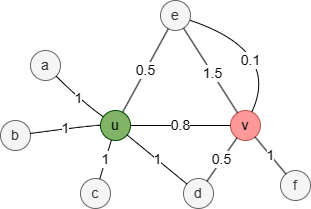
In this weighted graph, the union of the 1-hop neighborhood sets Nu and Nv is {a,b,c,d,e,f}. Set each element in the union set to the sum of the edge weights between the target node and the corresponding node, or 0 if there are no edges between them:
a | b | c | d | e | f | |
---|---|---|---|---|---|---|
N'u | 1 | 1 | 1 | 1 | 0.5 | 0 |
N'v | 0 | 0 | 0 | 0.5 | 1.5 + 0.1 =1.6 | 1 |
Therefore, the Weighted Jaccard Similarity between nodes u and v is (0+0+0+0.5+0.5+0) / (1+1+1+1+1.6+1) = 0.151515
.
Please ensure that the sum of the edge weights between the target node and the neighboring node is greater than or equal to 0.
Considerations
- The Jaccard Similarity algorithm ignores the direction of edges but calculates them as undirected edges.
- The Jaccard Similarity algorithm ignores any self-loop.
Example Graph
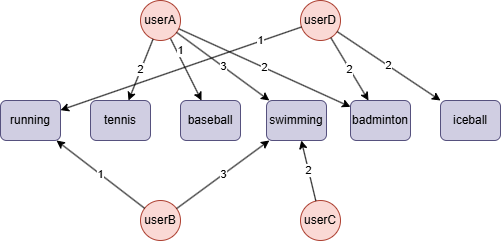
To create this graph:
// Runs each row separately in order in an empty graphset
create().node_schema("user").node_schema("sport").edge_schema("like")
create().edge_property(@like, "weight", int32)
insert().into(@user).nodes([{_id:"userA"}, {_id:"userB"}, {_id:"userC"}, {_id:"userD"}])
insert().into(@sport).nodes([{_id:"running"}, {_id:"tennis"}, {_id:"baseball"}, {_id:"swimming"}, {_id:"badminton"}, {_id:"iceball"}])
insert().into(@like).edges([{_from:"userA", _to:"tennis", weight:2}, {_from:"userA", _to:"baseball", weight:1}, {_from:"userA", _to:"swimming", weight:3}, {_from:"userA", _to:"badminton", weight:2}, {_from:"userB", _to:"running", weight:1}, {_from:"userB", _to:"swimming", weight:3}, {_from:"userC", _to:"swimming", weight:2}, {_from:"userD", _to:"running", weight:1}, {_from:"userD", _to:"badminton", weight:2}, {_from:"userD", _to:"iceball", weight:2}])
Creating HDC Graph
To load the entire graph to the HDC server hdc-server-1
as hdc_sim_nbr
:
CALL hdc.graph.create("hdc-server-1", "hdc_sim_nbr", {
nodes: {"*": ["*"]},
edges: {"*": ["*"]},
direction: "undirected",
load_id: true,
update: "static",
query: "query",
default: false
})
hdc.graph.create("hdc_sim_nbr", {
nodes: {"*": ["*"]},
edges: {"*": ["*"]},
direction: "undirected",
load_id: true,
update: "static",
query: "query",
default: false
}).to("hdc-server-1")
Parameters
Algorithm name: similarity
Name |
Type |
Spec |
Default |
Optional |
Description |
---|---|---|---|---|---|
ids |
[]_id |
/ | / | No | Specifies the first group of nodes for computation by their _id ; computes for all nodes if it is unset. |
uuids |
[]_uuid |
/ | / | No | Specifies the first group of nodes for computation by their _uuid ; computes for all nodes if it is unset. |
ids2 |
[]_id |
/ | / | Yes | Specifies the second group of nodes for computation by their _id ; computes for all nodes if it is unset. |
uuids2 |
[]_uuid |
/ | / | Yes | Specifies the second group of nodes for computation by their _uuid ; computes for all nodes if it is unset. |
type |
String | jaccard |
cosine |
No | Specifies the type of similarity to compute; for Jaccard Similarity, keep it as jaccard . |
edge_weight_property |
[]"<@schema.?><property> " |
/ | / | Yes | Numeric edge properties used as the edge weights, summing values across the specified properties; edges without the specified properties are ignored. |
return_id_uuid |
String | uuid , id , both |
uuid |
Yes | Includes _uuid , _id , or both to represent nodes in the results. |
order |
String | asc , desc |
/ | Yes | Sorts the results by similarity . |
limit |
Integer | ≥-1 | -1 |
Yes | Limits the number of results returned; -1 includes all results. |
top_limit |
Integer | ≥-1 | -1 |
Yes | Limits the number of results returned for each node specified with ids /uuids in selection mode; -1 includes all results with a similarity greater than 0. This parameter is invalid in pairing mode. |
The algorithm has two calculation modes:
- Pairing: When both
ids
/uuids
andids2
/uuids2
are configured, each node inids
/uuids
is paired with each node inids2
/uuids2
(excluding self-pairing), and pairwise similarities are computed. - Selection: When only
ids
/uuids
is configured, pairwise similarities are computed between each target node and all other nodes in the graph. The results include all or a limited number of nodes with a similarity > 0 to the target node, ordered in descending similarity.
File Writeback
CALL algo.similarity.write("hdc_sim_nbr", {
params: {
return_id_uuid: "id",
ids: "userC",
ids2: ["userA", "userB", "userD"],
type: "jaccard"
},
return_params: {
file: {
filename: "jaccard"
}
}
})
algo(similarity).params({
projection: "hdc_sim_nbr",
return_id_uuid: "id",
ids: "userC",
ids2: ["userA", "userB", "userD"],
type: "jaccard"
}).write({
file: {
filename: "jaccard"
}
})
Result:
_id1,_id2,similarity
userC,userA,0.25
userC,userB,0.5
userC,userD,0
Full Return
CALL algo.similarity("hdc_sim_nbr", {
params: {
return_id_uuid: "id",
ids: ["userA","userB"],
ids2: ["userB","userC","userD"],
type: "jaccard"
},
return_params: {}
}) YIELD jacc
RETURN jacc
exec{
algo(similarity).params({
return_id_uuid: "id",
ids: ["userA","userB"],
ids2: ["userB","userC","userD"],
type: "jaccard"
}) as jacc
return jacc
} on hdc_sim_nbr
Result:
_id1 | _id2 | similarity |
---|---|---|
userA | userB | 0.2 |
userA | userC | 0.25 |
userA | userD | 0.166667 |
userB | userC | 0.5 |
userB | userD | 0.25 |
Stream Return
CALL algo.similarity("hdc_sim_nbr", {
params: {
return_id_uuid: "id",
ids: ["userA"],
type: "jaccard",
edge_weight_property: "weight",
top_limit: 2
},
return_params: {
stream: {}
}
}) YIELD jacc
RETURN jacc
exec{
algo(similarity).params({
return_id_uuid: "id",
ids: ["userA"],
type: "jaccard",
edge_weight_property: "weight",
top_limit: 2
}).stream() as jacc
return jacc
} on hdc_sim_nbr
Result:
_id1 | _id2 | similarity |
---|---|---|
userA | userB | 0.333333 |
userA | userC | 0.25 |