Overview
The Leiden is a community detection algorithm designed to maximize the modularity. It was developed to address potential issues of the popular Louvain algorithm, which can sometimes produce internally poorly connected or even disconnected communities. Meanwhile, the Leiden algorithm is faster than Louvain. This algorithm is named after Leiden, the city where its authors developed it.
References:
- V.A. Traag, L. Waltman, N.J. van Eck, From Louvain to Leiden: guaranteeing well-connected communities (2019)
- V.A. Traag, P. Van Dooren, Y. Nesterov, Narrow scope for resolution-limit-free community detection (2011)
Concepts
Modularity
The concept of modularity is explained in the Louvain algorithm. The Leiden algorithm introduced a new resolution parameter γ
(gamma) into the modularity formula:
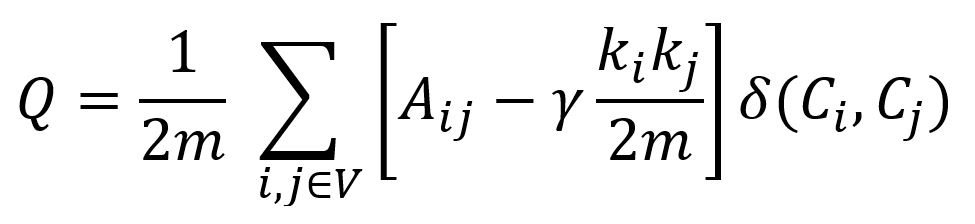
The parameter γ
modulates the density of connections within communities and between communities:
- When
γ
> 1, it leads to more, smaller and well-connected communities. - When 0 <
γ
< 1, it leads to fewer, larger and less well-connected communities.
Leiden
When the Leiden algorithm starts, each node is in its own community. Then algorithm iteratively runs through passes, and each pass is made of three phases:
First Phase: Fast Modularity Optimization
In the first phase of Louvain, it keeps visiting all nodes in the graph until no node movements can increase the modularity. The Leiden algorithm takes a more efficient approach. It only visits all nodes once, afterwards it only visits nodes whose neighborhood has changed.
To do that, the Leiden algorithm maintains a queue, initializes it by adding all nodes in the graph in a random order, then repeats the following steps until the queue is empty:
- Remove the first node
v
from the front of the queue. - Reassign node
v
to a different communityC
which has the maximum gain of modularity (ΔQ
) or keepv
in its original community if there is no positive gain. - If
v
is moved to a new communityC
, add to the rear of the queue all neighbors ofv
that do not belong toC
and that are not in the queue.
Second Phase: Refinement
This phase gets a refined partition Prefined
of P
that is produced from the first phase. Prefined
is initially set to a singleton partition, in which each node in the original or aggregate graph is in its own community. Then it refines each community C ∈ P
as follows.
1. Find each node v ∈ C
that is well-connected within C
by this formula:
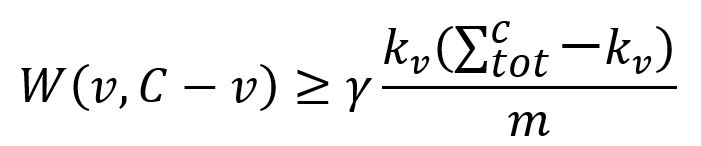
where,
W(v,C-v)
is the sum of edge weights between nodev
and nodes inC
.kv
is the sum of edge weights between nodev
nodes in the graph.is the sum of
k
of all nodes inC
.m
is the sum of all edge weights in the graph.
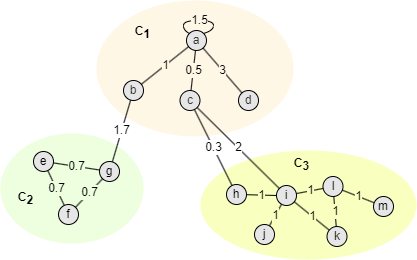
Take community C1
in the above graph as example, where
- m = 18.1
- = ka + kb + kc + kd = 6 + 2.7 + 2.8 + 3 = 14.5
Set γ
as 1.2, then:
- W(a, C1) - γ/m ⋅ ka ⋅ (
- ka) = 4.5 - 1.2/18.1*6*(14.5 - 6) = 1.12∑ tot C 1 - W(b, C1) - γ/m ⋅ kb ⋅ (
- kb) = 1 - 1.2/18.1*2.7*(14.5 - 2.7) = -1.11∑ tot C 1 - W(c, C1) - γ/m ⋅ kc ⋅ (
- kc) = 0.5 - 1.2/18.1*2.8*(14.5 - 2.8) = -1.67∑ tot C 1 - W(d, C1) - γ/m ⋅ kd ⋅ (
- kd) = 3 - 1.2/18.1*3*(14.5 - 3) = 0.71∑ tot C 1
Therefore, nodes a
and d
are considered well-connected in C1
.
2. Visit each node v
, if it is still on its own in Prefined
, randomly merge it to a community C' ∈ Prefined
for which the modularity increases. It is required that C'
must be well-connected with C
, which is judged by the formula below:

Note that each node v
is not necessarily greedily merged with the community that yields the maximum gain of modularity. However, the larger the gain, the more likely a community is to be selected. The degree of randomness in the selection of a community C'
is determined by a parameter θ
(theta) as:

Randomness in the selection of a community allows the partition space to be explored more broadly.
Third Phase: Community Aggregation
The aggregate graph is created based on Prefined
, and the aggregation process is the same as Louvain. Note that each node is a single community in the aggregate graph in Louvain. However, the aggregate graph in Leiden is partitioned based on P
, multiple nodes may belong to the same community.
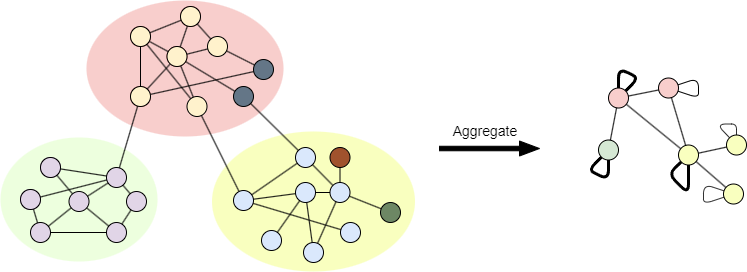
P
is denoted by color blocks, Prefined
is denoted by node colorsOnce this third phase is completed, another pass is applied to the aggregate graph. The passes are iterated until there are no more changes on nodes' communities, and a maximum modularity is attained.
Considerations
- If node
v
has any self-loop, when calculatingkv
, the weight of self-loop is counted only once. - The Leiden algorithm ignores the direction of edges.
Example Graph
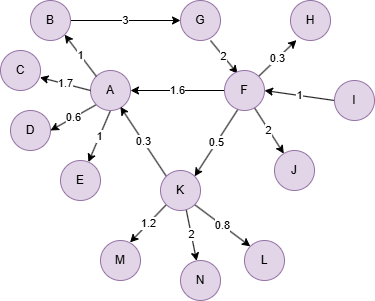
To create this graph:
// Runs each row separately in order in an empty graphset
create().edge_property(@default, "weight", float)
insert().into(@default).nodes([{_id:"A"}, {_id:"B"}, {_id:"C"}, {_id:"D"}, {_id:"E"}, {_id:"F"}, {_id:"G"}, {_id:"H"},{_id:"I"},{_id:"J"},{_id:"K"},{_id:"L"},{_id:"M"},{_id:"N"}])
insert().into(@default).edges([{_from:"A", _to:"B", weight:1}, {_from:"A", _to:"C", weight:1.7}, {_from:"A", _to:"D", weight:0.6}, {_from:"A", _to:"E", weight:1}, {_from:"B", _to:"G", weight:3}, {_from:"F", _to:"A", weight:1.6}, {_from:"F", _to:"H", weight:0.3}, {_from:"F", _to:"J", weight:2}, {_from:"F", _to:"K", weight:0.5}, {_from:"G", _to:"F", weight:2}, {_from:"I", _to:"F", weight:1}, {_from:"K", _to:"A", weight:0.3}, {_from:"K", _to:"M", weight:1.2}, {_from:"K", _to:"N", weight:2}, {_from:"K", _to:"L", weight:0.8}])
Creating HDC Graph
To load the entire graph to the HDC server hdc-server-1
as hdc_leiden
:
CALL hdc.graph.create("hdc-server-1", "hdc_leiden", {
nodes: {"*": ["*"]},
edges: {"*": ["*"]},
direction: "undirected",
load_id: true,
update: "static",
query: "query",
default: false
})
hdc.graph.create("hdc_leiden", {
nodes: {"*": ["*"]},
edges: {"*": ["*"]},
direction: "undirected",
load_id: true,
update: "static",
query: "query",
default: false
}).to("hdc-server-1")
Parameters
Algorithm name: leiden
Name |
Type |
Spec |
Default |
Optional |
Description |
---|---|---|---|---|---|
phase1_loop_num |
Integer | ≥1 | 5 |
Yes | The maximum number of loops in the first phase during each pass. |
min_modularity_increase |
Float | [0,1] | 0.01 |
Yes | The minimum gain of modularity (ΔQ ) to move a node to another community. |
edge_schema_property |
[]"<@schema.?><property> " |
/ | / | Yes | Numeric edge properties used as weights, summing values across the specified properties; edges without this property are ignored. |
gamma |
Float | >0 | 1 | Yes | The resolution parameter γ . |
theta |
Float | ≥0 | 0.01 | Yes | The parameter θ which controls the degree of randomness during community merging in the second phase; sets to 0 to disable randomness to acquire the maximum gain of modularity (ΔQ ). |
return_id_uuid |
String | uuid , id , both |
uuid |
Yes | Includes _uuid , _id , or both to represent nodes in the results. |
limit |
Integer | ≥-1 | -1 |
Yes | Limits the number of results returned; -1 includes all results. |
order |
String | asc , desc |
/ | Yes | Sorts the results by count ; this option is only valid in Stream Return when mode is set to 2 . |
File Writeback
This algorithm can generate three files:
Spec |
Content |
---|---|
filename_community_id |
|
filename_ids |
|
filename_num |
|
CALL algo.leiden.write("hdc_leiden", {
params: {
return_id_uuid: "id",
phase1_loop_num: 5,
min_modularity_increase: 0.1,
edge_schema_property: 'weight'
},
return_params: {
file: {
filename_community_id: "f1.txt",
filename_ids: "f2.txt",
filename_num: "f3.txt"
}
}
})
algo(leiden).params({
projection: "hdc_leiden",
return_id_uuid: "id",
phase1_loop_num: 5,
min_modularity_increase: 0.1,
edge_schema_property: 'weight'
}).write({
file: {
filename_community_id: "f1.txt",
filename_ids: "f2.txt",
filename_num: "f3.txt"
}
})
Result:
_id,community_id
I,5
G,7
J,5
D,9
N,11
F,5
H,5
B,7
L,11
A,9
E,9
K,11
M,11
C,9
community_id,_ids
5,I;J;F;H;
7,G;B;
9,D;A;E;C;
11,N;L;K;M;
community_id,count
5,4
7,2
9,4
11,4
DB Writeback
Writes the community_id
values from the results to the specified node property. The property type is uint32
.
CALL algo.leiden.write("hdc_leiden", {
params: {
return_id_uuid: "id",
phase1_loop_num: 5,
min_modularity_increase: 0.1,
edge_schema_property: 'weight'
},
return_params: {
db: {
property: 'communityID'
}
}
})
algo(leiden).params({
projection: "hdc_leiden",
return_id_uuid: "id",
phase1_loop_num: 5,
min_modularity_increase: 0.1,
edge_schema_property: 'weight'
}).write({
db: {
property: 'communityID'
}
})
Stats Writeback
CALL algo.leiden.write("hdc_leiden", {
params: {
return_id_uuid: "id",
phase1_loop_num: 5,
min_modularity_increase: 0.1,
edge_schema_property: 'weight'
},
return_params: {
stats: {}
}
})
algo(leiden).params({
projection: "hdc_leiden",
return_id_uuid: "id",
phase1_loop_num: 5,
min_modularity_increase: 0.1,
edge_schema_property: 'weight'
}).write({
stats: {}
})
Result:
community_count | modularity |
---|---|
4 | 0.548490 |
Full Return
CALL algo.leiden("hdc_leiden", {
params: {
return_id_uuid: "id",
phase1_loop_num: 5,
min_modularity_increase: 0.1
},
return_params: {}
}) YIELD r
RETURN r
exec {
algo(leiden).params({
return_id_uuid: "id",
phase1_loop_num: 5,
min_modularity_increase: 0.1
}) as r
return r
} on hdc_leiden
Result:
_id | community_id |
---|---|
I | 5 |
G | 7 |
J | 5 |
D | 9 |
N | 11 |
F | 5 |
H | 5 |
B | 7 |
L | 11 |
A | 9 |
E | 9 |
K | 11 |
M | 11 |
C | 9 |
Stream Return
This Stream Return supports two modes:
Item | Spec | Columns |
---|---|---|
mode |
1 (Default) |
|
2 |
|
CALL algo.leiden("hdc_leiden", {
params: {
return_id_uuid: "id",
phase1_loop_num: 6,
min_modularity_increase: 0.1
},
return_params: {
stream: {}
}
}) YIELD r
RETURN r
exec{
algo(leiden).params({
return_id_uuid: "id",
phase1_loop_num: 6,
min_modularity_increase: 0.1
}).stream() as r
return r
} on hdc_leiden
Result:
_id | community_id |
---|---|
I | 5 |
G | 7 |
J | 5 |
D | 9 |
N | 11 |
F | 5 |
H | 5 |
B | 7 |
L | 11 |
A | 9 |
E | 9 |
K | 11 |
M | 11 |
C | 9 |
CALL algo.leiden("hdc_leiden", {
params: {
return_id_uuid: "id",
phase1_loop_num: 6,
min_modularity_increase: 0.1,
order: "asc"
},
return_params: {
stream: {
mode: 2
}
}
}) YIELD r
RETURN r
exec{
algo(leiden).params({
return_id_uuid: "id",
phase1_loop_num: 6,
min_modularity_increase: 0.1,
order: "asc"
}).stream({
mode: 2
}) as r
return r
} on hdc_leiden
Result:
community_id | count |
---|---|
7 | 2 |
5 | 4 |
9 | 4 |
11 | 4 |
Stats Return
CALL algo.leiden("hdc_leiden", {
params: {
return_id_uuid: "id",
phase1_loop_num: 6,
min_modularity_increase: 0.1
},
return_params: {
stats: {}
}
}) YIELD s
RETURN s
exec{
algo(leiden).params({
return_id_uuid: "id",
phase1_loop_num: 6,
min_modularity_increase: 0.1
}).stats() as s
return s
} on hdc_leiden
Result:
community_count | modularity |
---|---|
4 | 0.397778 |